Interval training has been used by athletes in practically all endurance sports for decades. In many cases, designing and interpreting such workouts with respect to their specific physiological demands is relatively straightforward. For example, constant-intensity efforts of, say, 3-8 min duration with a work:rest ratio of 1:0.5 to 1:2 would, if done at an adequate intensity, be expected to place significant strain upon the O2 transport system, and thus contribute to an improvement in VO2max. At the same time, however, it is unlikely that any individual could repeatedly perform efforts of this duration at a high enough intensity to result in truly marked improvement in anaerobic capacity (although there might be some increase).
While the physiological demands of workouts similar to the one described above are fairly easy to understand, cyclists often perform interval sessions that are far more complex in nature, and thus much more difficult to interpret. For example, the work:rest ratio is often manipulated to try to mimic the demands of competition, i.e., to prepare for the seemingly stochastic nature of racing. In addition, most cyclists perform their interval sessions on the open road, where the terrain may vary, versus on a flat track as, e.g., runners tend to do. Finally, the higher speeds achievable by cyclists vs. runners (or swimmers) means that they often accelerate “violently” at the start of intervals, which at least has the potential to result in significant neuromuscular demands not found with steadier efforts, or those that begin with more of a rolling or flying start. Examples of the sorts of training sessions that would fall into this 2nd category include the 30 s on, 30 s off (or 40 s on, 20 s) off intervals often used to simulate the changes in pace that occur in criteriums or when racing off-road, ladder sessions such as the 1 min – 2 min – 3 min – 4 min – 4 min – 3 min – 2 min – 1 min intervals described by Joe Friel or the 1500 m descending to 100 m session popularized by Eddie Borysewicz, etc.
In our book we provide guidelines (via the training levels) for appropriate power ranges to target when doing different types of intervals, and also discuss how to pace such intervals as well as when to stop doing them (i.e., the intervals-to-exhaustion concept). These guidelines and suggestions, however, are most useful when applied to “plain vanilla” intervals workouts such as described in the 1st paragraph above, and don’t always tell the fully story when it comes to the more complex sorts of training sessions described in the 2nd paragraph. What I would like to discuss here is how you can gain deeper insight into the specific demands of such interval workouts by analyzing them to answer three questions:
What was the power after smoothing the data using a 30 s rolling average?
The physiological responses to exercise follow a characteristic time course, many of which are directly or indirectly related to the kinetics of changes in the rate of mitochondrial respiration in muscle at the onset and offset of exercise. Thus, smoothing of the power data using a 30 s rolling average can be used to provide an approximation of the time course of changes in muscle, and hence whole-body, VO2, and hence aid in understanding the metabolic and cardiorespiratory demands of a given training session. (An exponentially-weighted moving average would theoretically provide an even better estimate of changes in VO2, but even that would be an oversimplification due to intensity- and duration/fatigue-related changes in O2 demand and kinetics.)
For example, if some particular combination of work and rest periods and power outputs results in a smoothed power output that approaches or exceeds perhaps 110% of the individual’s functional threshold power for several minutes at a time, then that workout likely represents a significant stimulus for adaptation in, or at least maintenance of, VO2max. On the other hand, if the work periods are of insufficient intensity or duration and/or the rest periods between them are so easy and/or so long that this is not true, then this will not be true – in that case, there is much less assurance that the individual’s maximal aerobic capacity has been significantly stressed, and some other aspect of physiological function, e.g., neuromuscular power or anaerobic capacity must have been primarily “targeted”. Indeed, if the smoothed power significantly exceeds approximately 120% of functional threshold power for any significant duration, then the athlete’s anaerobic capacity has almost certainly been stressed to at least some degree, since in most cyclists 120% of functional threshold power approximates power at VO2max, and VO2max sets the upper limit to aerobic energy production. Improvements in anaerobic capacity may also be stimulated by repeatedly incurring a large O2 deficit at the onset of each interval, which would be apparent from a significant difference in the areas under the curves of the actual and smoothed powers during the early portion of each effort.
What does a quadrant analysis show?
Examining the smoothed power from a series of intervals can provide insight into the metabolic and cardiovascular demands of the workout, as described above. To fully understand the neuromuscular demands, however, it is often useful to perform a quadrant analysis, i.e., to calculate the average effective pedal force (AEPF) and circumferential pedal velocity (CPV) used to generate the power and plot them against each other. Doing so provides an easy way of appreciating the effects of, e.g., starting each interval with a hard jump in a large gear from a slow speed versus just rolling into the start of each effort in a more moderate gear, or performing intervals on a hill versus on the flats. Quadrant analysis is especially helpful in understanding how use of very short work and rest periods (i.e., performing “microintervals”) can at least partially dissociate the metabolic/cardiorespiratory and neuromuscular demands, allowing greater emphasis on the latter than would otherwise occur.
Less obviously, quadrant analysis also provides an effective (albeit clearly not only) means of quickly understanding what the rider did during the rest periods. In particular, less experienced or less well-trained cyclists often experience the overwhelming urge to stop pedaling entirely after a strenuous effort. If so, there will be an absence of, or at least a relative paucity of, points in quadrants III and IV (since when cadence and hence CPV is zero, AEPF is undefined). Except when descending, though, it isn’t often that you get to coast for any extended period of time when racing. Continuing to “soft pedal” during each rest period may therefore not only better mimic the demands of actual competition, but could also enhance recovery from a physiological perspective, e.g., by increasing clearance of lactate, by activating the muscle pump, etc. Coaches therefore may (or may not) wish to encourage their athletes to pedal easily instead of coasting between intervals, with quadrant analysis providing a quick-and-easy way of visualizing whether this instruction was followed.
What was the normalized power for the entire session?
The normalized power algorithm is intended to be a predictor of physiological strain, not the adaptation(s) resulting from that strain. As such, the actual power really says more about the specific adaptations likely to result from a particular interval workout, especially when the data are smoothed in a physiologically-relevant manner as described above. Nonetheless, examining the normalized power for an interval training session can often be enlightening. Specifically, if the normalized power for a series of intervals (across all sets, and including the final rest period) lasting more than 20 min significantly exceeds the individual’s well-established mean maximal power curve for that duration, this implies that either:
1) their fitness has improved,
2) their power meter was miscalibrated, or
3) they have generated an “NP buster”.
While performing a series of interval-like efforts is one way of “breaking” the normalized power algorithm, this is actually quite difficult to do, especially when considering longer workouts. Calculating the normalized power for a series of intervals therefore provides a way of quickly ascertaining whether either of the first 1st possibilities listed above may hold true. In addition, the normalized power algorithm provides a good “sanity check” when designing new intervals sessions, i.e., if the normalized power over the entire series of work and rest periods exceeds a rider’s mean maximal power curve, it is highly unlikely that they will be able to complete the workout as planned. (Indeed, I have previously posted a list of theoretically-impossible intervals workouts on the wattage list here: http://groups.google.com/group/wattage/msg/22bd6beb194270eb and only one person reported that they were able to meet the challenge I put forth.) Conversely, if based on the normalized power (and the rider’s functional threshold power) the workout should be “doable” yet the rider still must cut the workout short, then this provides evidence that something else is amiss, e.g., they have not recovered adequately from prior training sessions, they are getting ill, their motivation was abnormally low due to outside stressors, etc.
Analyzing interval workouts using power data: a case study of 30 s on, 30 s off intervals
To help illustrate the ideas presented above, I analyzed an interval workout performed by another cyclist that consisted (after a ~30 min warm-up) of 20 x 30 s on, 30 s off, during which the rider targeted 370 W (~125% of their functional threshold power) during the work periods and 250 W (~85% of their functional threshold power) during the rest periods. Figure 1 below shows the actual power data during the intervals both without and with smoothing using a 30 s rolling average:
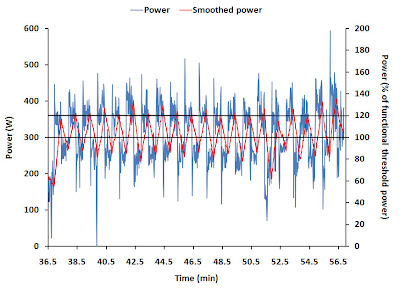
Although the workout in question is not likely to have contributed to any improvement in the rider’s anaerobic capacity, it did entail a moderate neuromuscular demand, as evidenced by the quadrant analysis plot shown in Figure 2 below:
Figure 2. Quadrant analysis of an interval training session consisting of 20 x 30 s on, 30 s off
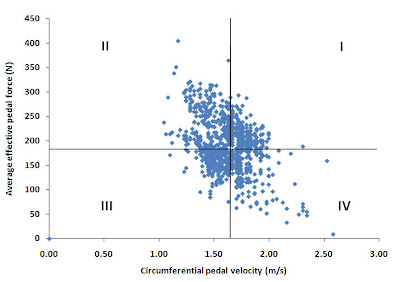
As shown in the figure, the rider spent a significant portion of their time (56%, to be exact) in quadrants I and II during the intervals, implying that significant recruitment of type II, or fast-twitch, muscle fibers must have occurred. This was the result of not only the rider’s supra-functional threshold power during each work period, but also the fact that they initiated each 30 s effort from a cadence somewhat below their preferred cadence when time-trialing. Whether their AEPF represented a sufficient overload to improve their maximal neuromuscular power could only really be determined by comparing the data from this interval session to their maximal AEPF-CPV relationship. At a minimum, however, it is apparent that the intervals were effective in replicating the frequent, moderately high power accelerations that occur during mass start races, e.g., when exiting a sharp turn.
In summary, in this article I have described how to analyze power data from interval training sessions to gain deeper insight into their true physiological demands, and hence the nature of the adaptation(s) they are likely to produce. Applied to a typical 30 s, 30 s off series of intervals, the approach demonstrates why such intervals are so commonly used to prepare for the constant, “on-off” nature of criterium racing, which tend to place greater emphasis on neuromuscular power (e.g., jumping out of turns, launching attacks, sprinting at the finish) and VO2max (e.g., chasing down breakaways) than on anaerobic capacity or functional threshold power, both of which are called upon during more extended efforts of somewhat lower intensity than typically occur during criteriums. Indeed, this is reflected in the ‘\/\’ power profile found in many riders who tend to focus on/excel in such events (or who do a lot of unstructured endurance training combined with group rides and races). This is not to say, of course, that these are the perfect way of preparing for such races – rather, the point is that the approach I have described can often be profitably used to analyze interval workouts sessions of all types, so that training sessions are appropriately prescribed to meet the demands of any event.